Examining the Relationship Between Expected Value and Variance in Dependent Variables
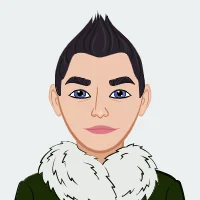
Students often face assignments in statistics that require a deep understanding of core concepts and the ability to apply them effectively to solve complex problems. One particularly challenging area is the calculation of expected value (mean) and variance when dealing with the sum and difference of two dependent random variables. These problems demand not only a solid grasp of basic statistical principles but also the ability to navigate the intricacies of relationships between variables that are not independent. Successfully approaching these tasks requires a systematic method that breaks down the problem into manageable steps, allowing students to address each part with clarity and confidence.
Focusing on the specific example of expected value and variance calculations, the strategies outlined provide a robust framework applicable to a wide range of statistical challenges. By mastering these techniques, students can develop a deeper understanding of how to solve your statistics homework and effectively address similar assignments, irrespective of the specific details. This approach, which integrates both theoretical and practical elements, not only supports the completion of current coursework but also establishes a solid foundation for future statistical work, thereby enhancing both academic performance and practical problem-solving skills.
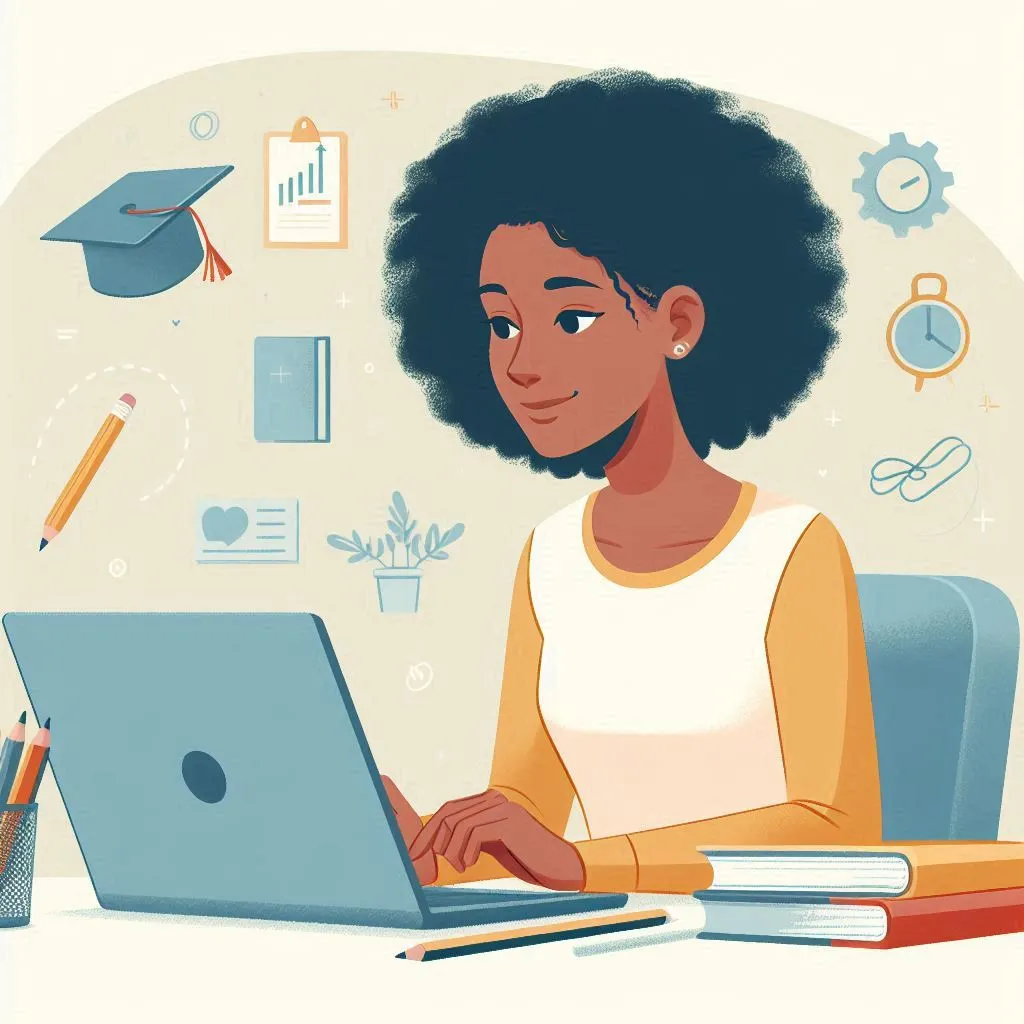
Understanding Dependent Random Variables
Random variables play a crucial role in statistics, and understanding their relationship is key to solving problems involving them. Dependent random variables are those whose outcomes are not independent of each other. This means that the occurrence of one event affects the probability of the other. The covariance between two random variables is a measure of their dependence. A positive covariance indicates that the variables tend to move in the same direction, while a negative covariance suggests they move in opposite directions. Mastery of this concept is essential for solving problems involving the sum and difference of dependent random variables.
Dependent random variables often appear in real-world scenarios, such as in finance, where the performance of two stocks might be correlated. Understanding this dependency is crucial when calculating combined metrics like portfolio returns or risk assessments. For example, in a finance assignment, you might be required to calculate the expected return of a portfolio composed of two assets. If these assets are dependent, their covariance must be considered to accurately determine the portfolio's expected return and risk.
To tackle such assignments effectively, start by clearly identifying the random variables and understanding their relationship. Assess whether the variables are dependent by evaluating their covariance. If covariance is not provided directly, you may need to calculate it using the appropriate formula. Covariance represents the expected value of the product of the deviations of the two variables from their respective means. For those seeking professional help with Statistics homework, grasping and calculating covariance is essential for accurately handling the sum and difference of dependent random variables. Once the covariance is determined, proceed with calculating the sum and difference of the random variables, ensuring to account for their interdependence.
Calculating Expected Value (Mean) for Sum and Difference
The expected value, or mean, of a random variable provides a measure of the central tendency or average outcome. When dealing with the sum and difference of two dependent random variables, the expected values can be computed using the linearity of expectation. Specifically, the expected value of a sum is the sum of the expected values, and the expected value of a difference is the difference of the expected values. Understanding this property simplifies the process of finding the mean for complex random variables and forms the foundation for more advanced calculations.
The linearity of expectation is a powerful tool that can greatly simplify calculations. For instance, if you are given the expected values of two random variables, you can easily find the expected value of the sum and difference by simply adding or subtracting these values. This property holds true regardless of whether the variables are dependent or independent, making it a versatile approach in various scenarios. Applying this concept in your assignments will allow you to efficiently determine the expected values without needing to delve into more complex computations.
However, it’s important to ensure that you accurately interpret the context of the problem. For example, in an economics assignment where one variable represents demand and another represents supply, understanding the expected values can provide insights into market equilibrium. Misinterpreting the expected values could lead to incorrect conclusions about market dynamics.
Deriving the Variance for Sum and Difference
Variance is a measure of the spread or dispersion of a random variable's possible values. For dependent random variables, the variance of the sum and difference requires careful consideration of the covariance. The variance of the sum and the variance of the difference can be derived by applying the formula for the variance of a linear combination of random variables. This involves not only the variances of the two variables but also their covariance. Grasping this concept allows students to accurately assess the variability of combined random variables.
The variance of the sum and difference of two dependent random variables is impacted by their covariance. If the variables are positively correlated, the variance of their sum will be greater than the sum of their individual variances. Conversely, if they are negatively correlated, the variance of their sum may be less. This principle is crucial for understanding how the relationship between variables affects overall variability.
In practical terms, consider a scenario where you are analyzing the performance of two financial assets. If the assets are positively correlated, the risk (as measured by variance) of holding both assets together will increase. On the other hand, if the assets are negatively correlated, combining them could reduce overall risk. Being able to determine and interpret these variances is essential for making informed decisions in both academic and real-world contexts.
Practical Application of Covariance in Problem Solving
Covariance is a fundamental concept that often appears in statistics assignments involving multiple random variables. When solving problems related to the sum and difference of dependent variables, understanding how to manipulate covariance is crucial. For instance, the variance of the sum or difference incorporates the covariance term, reflecting the degree of dependence between the variables. Being able to identify and apply the correct covariance in different contexts is a skill that will significantly enhance a student's problem-solving abilities in statistics.
In real-world applications, covariance is used to assess the relationship between variables in fields such as finance, economics, and engineering. For example, in finance, covariance between different assets helps in constructing a diversified portfolio that minimizes risk. In an assignment, you might be required to calculate the overall variance of a portfolio and understand how the covariance between assets impacts the total risk.
When approaching such problems, start by understanding the context in which the covariance is applied. Ensure that you correctly identify the dependent variables and their relationship. Once you have a clear understanding of the covariance and how it interacts with variance, you can confidently apply this knowledge to solve complex problems.
Strategies for Simplifying Complex Calculations
Statistics assignments often involve intricate calculations that can be daunting at first glance. However, with the right strategies, these problems can be simplified. One effective approach is to break down the problem into smaller, more manageable parts. For example, when calculating the variance of a sum or difference, start by finding the variance of each individual variable and the covariance, then combine these results according to the relevant formulas. Additionally, practicing algebraic manipulation and being comfortable with the properties of expectation and variance can make the calculations more straightforward.
Breaking down complex problems into smaller steps not only makes the task more manageable but also helps you avoid errors. For instance, if you are asked to calculate the variance of a combined random variable, begin by calculating the variance of each variable separately. Next, determine the covariance, and finally, combine these components to find the overall variance. This step-by-step approach ensures accuracy and reduces the likelihood of mistakes.
Moreover, practicing these calculations regularly will help you become more familiar with the process, making it easier to solve similar problems in the future. Understanding the underlying concepts, such as linearity of expectation and the role of covariance, is crucial for simplifying complex statistical problems.
Real-World Applications and Interpretation of Results
Understanding the theory behind expected value and variance is only part of the equation; being able to interpret and apply these concepts in real-world scenarios is equally important. For instance, in finance, the sum and difference of dependent random variables might represent the total return and risk difference between two assets. Accurately calculating and interpreting these values can inform investment decisions. By linking theoretical knowledge to practical applications, students can better grasp the importance of these concepts and their relevance to various fields.
In assignments, you might be required to interpret the results of your calculations in the context of a specific problem. For example, after calculating the expected value and variance of a portfolio, you may need to explain what these results mean in terms of risk and return. Understanding how to interpret these results in a practical context is crucial for making informed decisions.
Moreover, real-world applications of these concepts extend beyond finance. In engineering, for example, understanding the variance of measurements can be critical for quality control. In economics, interpreting the covariance between variables can provide insights into market trends and inform policy decisions. By practicing the application of these concepts in various contexts, you will develop a deeper understanding of their significance and become more adept at solving complex problems.
Common Mistakes and How to Avoid Them
Statistics assignments can be challenging, and it's easy to make mistakes, especially when dealing with dependent random variables. Common errors include incorrect application of the covariance term, misunderstanding the properties of expectation and variance, and overlooking the dependence between variables. To avoid these pitfalls, students should carefully review the problem, ensure they understand the relationships between the variables, and double-check their calculations. Practice and familiarity with similar problems can also help minimize errors and improve accuracy.
One common mistake is neglecting to account for the covariance when calculating the variance of a sum or difference of dependent random variables. This oversight can lead to incorrect results and a misunderstanding of the overall variability. To avoid this, always remember to consider the covariance when dealing with dependent variables.
Another frequent error is misinterpreting the expected values or variances in the context of the problem. For example, incorrectly assuming that two variables are independent when they are actually dependent can lead to significant errors in your calculations. To prevent this, take the time to thoroughly understand the problem and the relationships between the variables before starting your calculations.
Finally, double-checking your work is crucial for catching any mistakes that might have occurred during the calculation process. By reviewing each step and ensuring that your reasoning is sound, you can minimize errors and improve the accuracy of your results.
Conclusion
Mastering statistics, particularly when dealing with the expected value and variance of dependent random variables, requires a strong grasp of fundamental concepts and the ability to apply them effectively. This guide has outlined a systematic approach to solving such problems by breaking down the key steps involved. From understanding the nature of dependent random variables to calculating their mean and variance, and applying these concepts to real-world scenarios, each section has provided valuable insights that can be applied to a wide range of statistics assignments.
By developing these skills and strategies, students will be better equipped to tackle complex statistical problems with confidence and accuracy. Whether you're working on a challenging assignment or preparing for an exam, the knowledge and techniques outlined in this guide will help you achieve success in your statistics coursework and beyond.